There is an inevitable change coming to all industries involved in geoscience analytics, be it sea wind, geothermal, Oil and gas or CCS. That change is pushed by the plateau we have reached with our traditional methods and technology. From 2011 to 2015, oil and gas companies in Norway invested a staggering 145 billion NOK (14.5 billion $) into 187 exploration wells, with fewer than 10% becoming commercially viable. As discoveries dwindle and market and industry demands rise, it's clear that a shift is urgently needed.
In any innovative industry, change is not just inevitable; it's a constant. Yet, this doesn't make the process of adopting new technologies and methods any less challenging.
Previous experiences with underwhelming solutions can foster a reluctance to venture into new technological territories. Many in the oil and gas industry might recall the “Bright Spot” technology of the 70s, AVO in the 80s, and later, CSEM. Each promised to revolutionise the industry but encountered significant challenges and shortcomings when brought to the test of practical implementation.
There's however a key distinction between the technological advances that unfolded before the turn of the century and what we see today. That distinction lies in our newfound recognition of the critical importance of data handling. In the past, there was an overarching focus on optimising the analytics process, often overlooking the true foundation of it all: the data itself.
The 5 Key Benefits of Implementing AI-Driven Workflows for Your Geoscience Team
Let's have a look at the 5 key benefits that we see from using AI workflows in your analytics work. Like a neural net, they are all connected, but are also stand-alone important factors of why you and your team should adopt this new, groundbreaking technology.
1. Data Liberation and Elimination of Tedious Tasks
The painstaking job of searching for, collecting and preparing the data usually tops the list of dull tasks for most geoscientists. These tasks start before a question or hypotheses has even been made, and includes:
- Locating data
- Organising it
- Selecting the relevant data
- Contextualising
- Cleaning
- Sub-setting
It’s one of the aspects of the job where “it was always like that”, but finally something new has come along. Since AI is so reliant on the data, a part of its implementation is solid data governance, which will lead to “data liberation” if properly planned and implemented.
Perhaps the second most mundane task a geoscientist can find in their career would be the immense undertaking of structuring and organising large data sets or big data. Making order out of the existing data-chaos, is a feature of machine learning models. This is at the core of data ingestion systems, like a data lake. Higher abstraction layers provide instant access to any data point. Taking the idea of meta data to the next level, it includes higher contextualisation, as revision history and enhanced schema definitions.
Where you once upon had to find the person who could say where you’ll find a certain set, you can now access any point instantly, and across multiple applications. This provides new opportunities for more meaningful exploration, at scale. Geoscientists core time has to be spent on working with data and not looking for data.
Getting your data in order has two key mechanisms:
- Centralisation
- Contextualisation
Data has traditionally been stored in silos, being application-specific for the task at hand. It is also never “all the data”, but manageable sub-sets. The OSDU initiative is a good foundation of centralising your data. It is not locked to proprietary technologies, allowing you an application-neutral data layer accessed through APIs. With a solid community and documentation, OSDU and Open Data Layer is accessible, and already adopted by many key industry actors. this is only the beginning. Data lake is the continuation.
Proper alignment, contextualisation and cleaning of the data, and building consistent subsurface data is essential for ML applications. You now have instant access to any data-point, of entire datasets. There is some human input required in the process, but once it is done, it is done. Now, new opportunities will emerge, that has until now been unobtainable.
2. Enhanced Efficiency (No Waiting Game)
In Geoscience analytics, there are complex problems being solved based on incredible amounts of data. Therefore, our current methods rely on a relay-race approach. The baton are the rules, definitions and predictions made within each field of expertise, which are passed on to the next subject matter expert. With integrated AI workflows, this is all connected and to a large degree automated.
With downtime coming at a million-dollar-a-day premium, waiting for predictions, or the next piece of the puzzle from your peers, is stressful. And nobody but our established methodologies are to blame. Evolving our approach, not only relieves pressure for the individual, now able to better spend their time. It also allows the entire organisation to increase its throughput, exponentially, through its employees.
3. Expand Your Own Areas of Expertise
As fascinating as new technologies can be on an academic level, many will be ambivalent when looking up at a learning curve. Furthermore, why absorb a new technique when having mastered the one already?
We believe new technology must be easy to use to gain adoption. There were a lot of quirky touchscreen devices before Apple released their first iPhone, changing the whole industry. Understanding technology from their users’ point of view, not the underpinning technology, is key to bringing any kind of change. With a solid and frictionless UI and UX, you can build upon your existing knowledge and understanding. This way, new opportunities and even fields of study will emerge.
4. Endless Possibilities With a More Contextualised Overview
It's only when you have proper context, that you can ask the right questions. Machines can’t ask questions. They can only provide answers. Furthermore, asking questions without all the data or context of that data, then your answer will also be inconclusive. As the saying goes, rubbish in, rubbish out.
Mixing data from 60 years ago with that collected in 2023 is unheard of. However, that is about to change. With instant access to any data-point across decades of data, cleaned and contextualised by ML models, you can ask more productive and meaningful questions. No longer are you bound by the relay race. A hypothesis can be formed, and tested, without the mundane tasks of data acquisition and preliminary validation. Go straight to your problem, and spend your time on good questions, rather than potentially incomplete answers.
5. Simply the Only Way of Approaching Big Data
The amount of data we are collecting is growing exponentially. The amount of data generated and stored are doubling roughly every other year. Fidelity of instruments improve, transfer protocols, compression and data-structure are becoming more sophisticated, while storage mediums are becoming larger and cheaper and data transfer is becoming faster. There was already too much data for anyone to process in a lifetime, hence our methods of sub-setting data and the relay-race approach to product predictions.
AI workflows will have the impact on the sciences, comparable to that of computers in the 50s. Some even claim it is the most important thing since paper in analytics work. There is finally exists “a machine” that can understand and sift through data in record time, at least if it had the right instructors (read: developers). It has for a long time only been a tool within the big data of consumerism, but its applications are limitless if applied correctly.
Conclusion
There is a need to optimise the process of geoscience analytics, and AI workflows are the mean to push passed the barriers we are facing today. But there will always be resistance to new methods and technologies.
Elevating geoscience analytics above the plateau it has reached, require adoption of new tools by the subject matter experts in the forefront. The five benefits of AI outlined in this article is a part of that, as AI/ML technologies stand to become the new norm in geoscience analytics and data sciences in general.
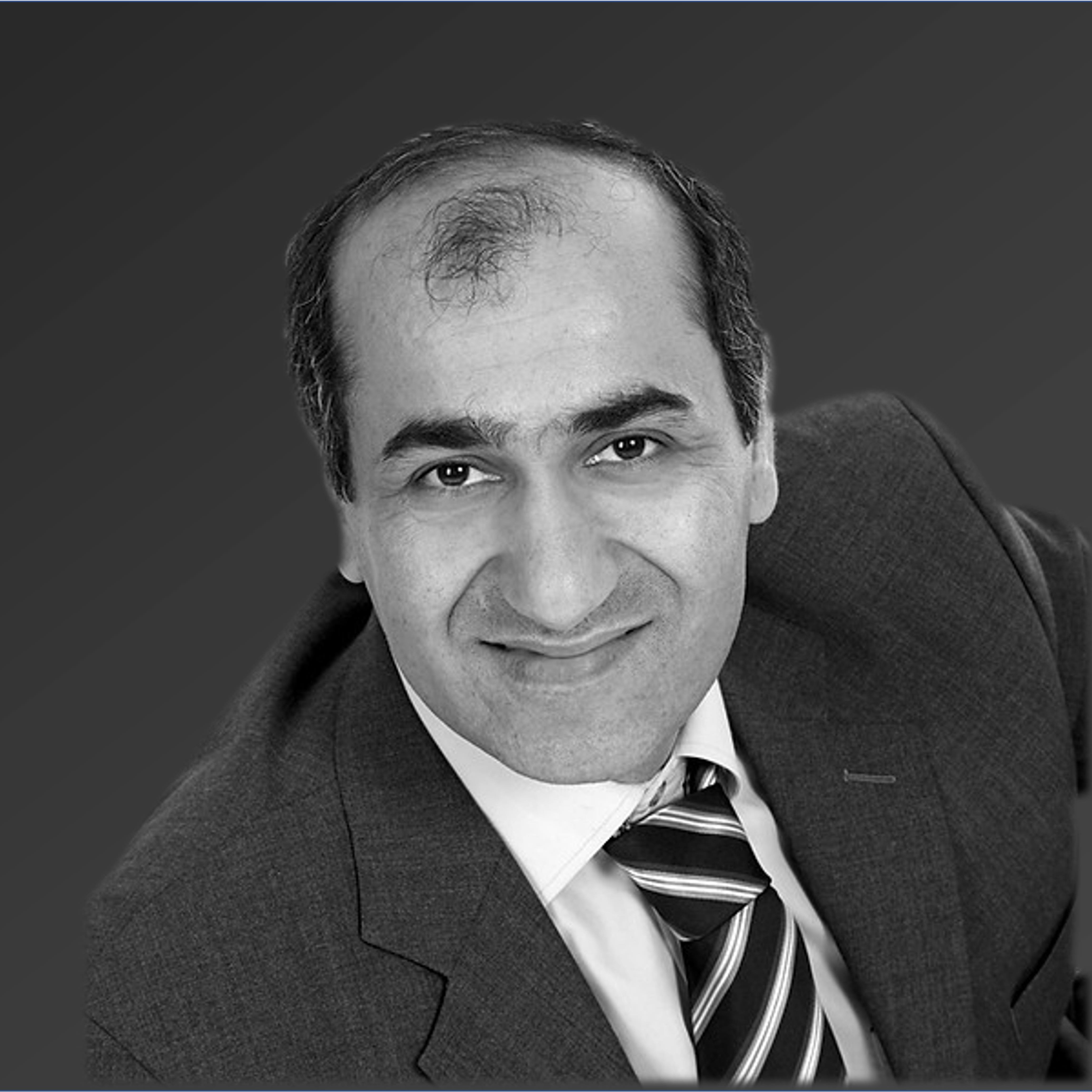