A pillar of an oil and gas exploration company rests on the number of prospects in their portfolio. Defining prospects and filling up the prospect portfolios can take time and resources; however, Machine Learning (ML)-driven workflows, where hydrocarbon pay prediction at well scale and reservoir properties (lithology, porosity and SW) at seismic scale is performed, can accelerate the prospect definition process when guided by geoscience domain experts.
This article will look at the how ML-driven workflows, when leveraged by ML experts and geoscientists, can lead to finding overlooked hydrocarbon pay in wells and compartments of hydrocarbons adjacent to existing producing assets in brown field exploration areas and around existing producing assets.
Next obvious step
ML-driven workflows are data thirsty and well suited to leveraging the large amounts of data residing in oil companies’ databases, especially in explorationally mature areas. ML-driven workflows are the next obvious step oil companies are pursuing to extract even more information from their data, providing a much-needed alternative and complimentary interpretation to existing work.
Data Silos
One of the major challenges facing oil companies is that data commonly resides in multiple databases and that don’t necessarily communicate with each other, i.e. data silos. The data silo situation does not promote finding inter-relationships between data types and hence has limited value. Value creation is gained by contextualising the data into one platform.
ML the accelerator
Traditionally defining hydrocarbon pay in a well depended on the work of a petrophysicist. However, limited time and resources means that this type of work is not able to be performed at scale. Hence, assessing hydrocarbon pay in many thousands of wells in a basin is just not realistic. ML-driven workflow can rapidly and accurately predict hydrocarbon pay in many thousands of wells in a consistent manner.
Prospect definition can be a stepwise process that is time consuming. It is commonly characterised by a workflow including creating GDE maps based on well and seismic data, mapping specific key horizons, attribute extraction, rock physics and quantitative interpretation methodologies, and hydrocarbon charge assessment. Much of this information can be gained rapidly and accurately using ML-driven workflows through:
- Automated seismic interpretation
- Geobody and fault extraction
- Reservoir properties and hydrocarbon pay mapping at well and seismic scales
ML-driven workflows are a much-needed accelerator to hydrocarbon exploration and exploitation in the fast-moving environment prevailing today.
Rubbish in = rubbish out
The results from ML-driven workflows are only as good as the quality of the training data, the saying of “rubbish-in equals rubbish out” is very prevalent in all ML studies. Quality controlling large datasets can be a daunting task, but methodologies are at hand to ensure the best quality data is obtained within a reasonable time frame. Data quality control is considered an important step along the ML journey.
Models making geological sense
Model creation and especially quality controlling the models is important as the models are required to be geologically viable. The results from the models must make geological sense. The domain experts, backed by years of knowledge and experience, can rapidly and intuitively assess the model’s accuracy to predict a geological feature. Again, human expertise is imperative to assess the geological significance of the model results.
Domain experts have a voice at the table
The rejuvenation of prospect portfolios is a challenge for all oil companies alike. ML-driven workflows can aid and accelerate the process of finding hydrocarbons whilst guided by domain experts. Domain experts, such as exploration and reservoir geologists/geophysicists, and petrophysicists, are important guides and decision makers in the ML-driven workflows, as their knowledge is vital to quality control data and models.
The advantages of pursuing a ML-driven workflow to finding hydrocarbons and rejuvenating prospect portfolios are:
- Qualified human resources, such as geologists and petrophysicist, are diminishing in number as many are leaving the industry and/or moving to new emerging markets in the energy transition domain.
- Workloads remain high in a sector where personnel and competence are leaving to pursue energy transition paths.
- Time required for a human to perform hydrocarbon pay identification on 1000s of wells is unrealistic in the current race of new prospects.
- The process of doing such analysis is time and labor intensive.
The challenge
It is the endeavour of all geoscientists to utilise and maximise the amount of information from all types of data. The search for inter-relationships between different diverse data types is key in their pursuit of finding hydrocarbons in the subsurface. There are huge challenges in processing and integrating the incredible amounts of geological data available using traditional methods.
Applying ML-driven workflows are now accessible alternatives and have been shown to deliver hydrocarbon pay prediction in many 1000s of wells and define prospects at seismic scale. This is the much-needed accelerator the industry has been waiting for in order to extract additional information from data residing in the oil company's databases.
New ML technologies are disruptive, creating new markets and opportunities. Being the only way to handle big data on a meaningful scale, it will have the same impact on scientific analysis work, as the internet has had on commerce since the 90s.
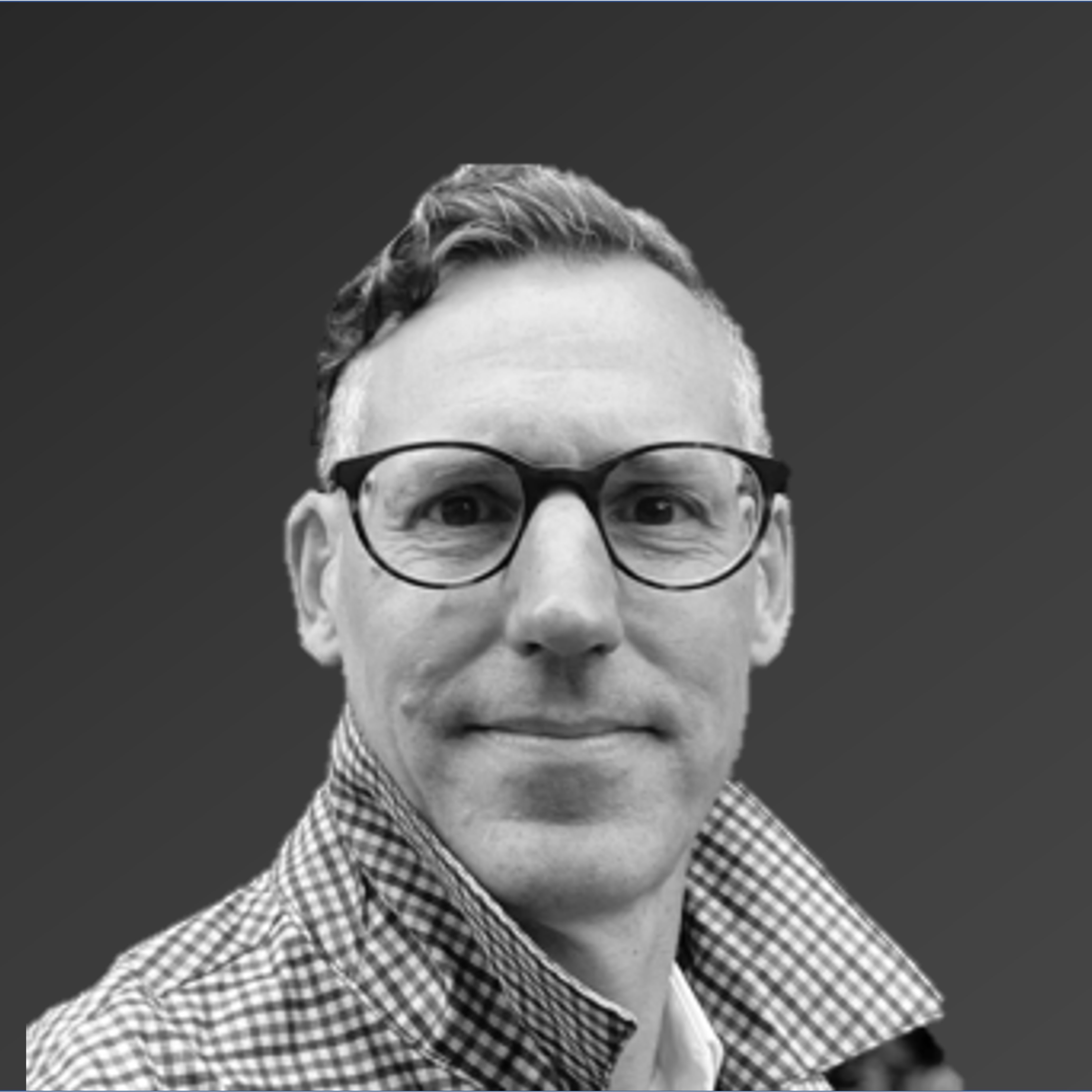